Market research to investigate the uptake and attitudes towards AI audio technologies
I conducted this research early during the discovery phase of the project to help inform product and business strategy. This research aimed to identify trends in consumer behaviour and gather insights into users' attitudes and experiences using AI-assisted equalisers and "Smart " audio effects.
My Role
-
Plan and run research: I defined the experimental design and prepared the research material for a quantitative survey with one open-ended question.
-
Data analysis: I structured, analysed, and visualised the resulting data. I performed hypotheses testing using analysis of variance on the aligned transformed data. I used thematic analysis.
-
Communicated Results: Presented research insights and design recommendations to product stakeholders.
Results
-
Assessed uptake of AI-assisted EQ and consumers' attitudes towards AI-audio effects.
-
Help understand consumers' perceptions of AI audio effects.
-
Identify key adoption drivers of AI technology in music production.
-
Made design recommendations.
-
Defined direction for future development of the software tool.
By assistive equalizers, I refer to AI-powered equalizers that analyze the audio input and use artificial intelligence to suggest EQ settings that help users improve the spectral balance, clean up unpleasant resonances and enhance the details of their sound.
Why conduct this survey research?
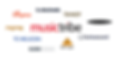
This market research aimed to answer several important questions for Music Tribe. Music Tribe has many different types of consumers, including hobbyists, musicians, live audio, and post-production professionals. The skills of those customers vary from novice to professional, and consequently, their needs differ too. Music Tribe owns several brands that produce products in various product categories, such as synths, microphones, speakers and audio effects. Furthermore, consumers might be experts in one domain but not in all realms of music production. Given that AI-augmented audio effects are relatively new, we don't yet know the needs and attitudes of different customer segments towards AI and how to use this technology to meet these needs effectively. Instead of second-guessing what customers want, we reached out to our customers to find out about their perceptions, attitudes and needs and minimise business risk.
The project
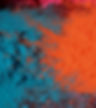
At MusicTribe, we wanted to know our customers' perceptions, attitudes, and past experiences using AI-assistive equalisers. Furthermore, we wanted to verify our assumptions about customer acceptance of AI technology by different audio market customer segments. We gathered insights from 261 consumers by inviting them to tell us about their experience using our competitors' tools and the reasons for adopting AI technology in the first place. We asked them about their general attitude towards AI in music production. The findings assisted in competitor analysis by providing insights into what users liked and disliked from their experience of using competitors' tools. Moreover, we got a sense of how ready the market is for this type of technology and the likelihood of different market segments adopting AI software tools, which have the potential to inform business and marketing strategies. Finally, the insights we gained are useful for informing the design of our AI-assistive equaliser.
What?
-
An online survey which was administered on Behringers’ Facebook page
-
This survey was part of a series of synergetic studies on AI-assisted equaliser
-
We aim to gather information about peoples’ attitudes and experiences of using AI-assisted equaliser
-
Identify patterns of user attitudes as a result of demographic and behavioural characteristics


-
What are peoples’ attitudes towards smart equalisers?
-
What are peoples’ experiences using smart equalisers?
-
Do subjects’ feelings, trust, and perceived product qualities vary as a result of their age, subjects’ skill type, and level?
-
What do subjects with experience using smartEQ like/dislike about them?
Research questions

Hypotheses
H1: Users' feelings, trust, and perceived quality of the smartEQ will vary as a result of their skill level and type.
More skilled and older people will
-
H1.1 have more negative feelings towards smartEQ and AI in general
-
H1.2 be less likely to trust smartEQ than less experienced users
-
H1.3 will not values the smartEQ assistance as much as less experienced users
H2: Irrespectively of participants' age and skill, users value the ability to correct and overwrite the AI suggestions
H3: Irrespectively of participants' age and skill, Users value contextual information that helps them understand the output of the smartEQ
Dependent variable

Using Likert Scales, we quantitatively assessed UX metrics that fall into three major categories: feelings, trust, and perceived product quality.
Feelings
-
Feeling more in control
-
Feeling less inadequate
-
Feeling more productive
-
Feeling more confident
Trust
-
Trust
-
Suspicion
Perceive product quality
-
Perceived usefulness
-
Perceived ease of use
-
Influence their decision about how to process sound
-
Perceived flexibility
We used open-ended questions asking the participants to help us interpret their preference for one interface over another.

Factors & Constant variables
Four between-subjects factors
Subject Age
Levels: 18-24, 25-34 ……….65 to older
Subject Skill
Levels: Creative, Musician, Engineer
Subject level of expertise
Levels: Amateur, Advanced, Professional
Whether subjects earn money from music
Levels: No, Some money, Significant income
Mixed linear model, no repeated measures using Analysis of Variance of Aligned Rank Transformed Data
260 Participants
In total, we collected 260 responses. Among the 260 participants, 101 participants had prior experience using an AI-Assistive equaliser, while the remaining 159 participants had never tried this type of technology. The figures below show participants' background data corresponding to the constant variables used for factor analysis of the Likert type data.




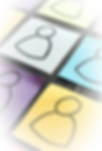
Study procedure
The list below explains the procedures of the study:
-
Welcome, Introduction & Consent pages
-
Participant screening: to take part in the survey, users had to engage in music-making or sound production
-
Participants who qualified took part in the survey.
-
Each participant was asked if they used an AI assistive equaliser:
-
If the participants had used an AI-assisted equaliser were then asked a series of questions aimed at measuring the participants' feelings, trust, and perceived product quality. Then participants were asked an open-ended question asking what they liked and/or disliked about the AI-assistive EQ they tried.
-
If participants had not used an AI-assisted equaliser, they were asked whether they would consider using one, followed by a series of questions to measure the respondents anticipated benefits of using an AI-assisted equaliser.
-
-
Post-survey, they were asked to fill out a questionnaire about their demographic information and attitudes towards AI. This information enabled us to compare user groups based on their demographics and attitudes towards AI and see if these might have influenced their answers.
The average duration of the survey was 4 minutes.

The study procedure is shown from left to right. A screening survey is presented to the participants to ensure they have some experience with the type of music production software we are interested in. Participants who passed the screening test (marked as blue profile icons) will be directed to the survey task. The presentation order of the questions was randomized to minimise the order effect.
Data analysis of the attitudes and experiences of 101 consumers who have used AI-assistited equalisers
The figures below show the overall distribution of the consumers' responses to the Likert type questions of the questionnaire.
1 = strongly disagree, 5 = strongly agree
Actual questions participants were asked:
-
Explainability = It is important to me to understand how the assistive equalizer derives the recommended parameter settings.
-
NPS= I would recommend assistive equalizer plugins to a friend or colleague.
-
Feeling more productive = I use assistive equalizers because it speeds up my workflow.
-
Ease of use = I think assistive equalizers are easy to use.
-
Behavioural intent = I think the equalizer' suggestions influence my decisions while equalizing sound.
-
Perceived usefulness = Assistive equalizers are very useful to me.
-
Feeling more confident = I feel more confident that the settings I have applied are appropriate when I use an assistive equalizer.
-
Trust = I trust the suggestions of assistive equalizers which I have tried.
-
SmartEQ performance = I found the assistive equalizer's suggestions to be very relevant for the sounds I process.
-
Feeling in control = I think the assistive equalizer increases the level of control I have over the settings that are being applied to process the sound.
For measuring consumers' perceptions based on the UX metrics, I perform multifactor analysis on the non-parametric Likert responses and measure statistical significance. I used the Aligned Rank Transform method to align the non-parametric Likert responses in order to perform a multifactor analysis for testing statistical significance. The Aligned Rank Transform relies on a preprocessing step that "aligns" data before applying averaged ranks, after that common factorial ANOVA analysis procedures can be used for hypothesis testing. The tables below show the findings of the results of the tests.
A general ANOVA model was computed to investigate the effect of the users' age, skill type, skill level, and income factors on the consumers' feelings, trust, and perceived product quality metrics reported by the participants. An asterisk and green highlight color (*) indicate statistical significance.

Post-hoc testing, blue highlighted fields in the image shows which factor levels contribute to the observed effects.

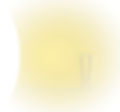
I performed thematic analysis on the optional (n=32 comments) open-ended question of the survey where participants responded to the question of what they liked or disliked about the AI-assisted equalisers they have tried. The list below shows the summary of the findings. The number indicates the number of participants who have referred to that topic. I have used the plus and minus symbols and color-coded (red=nagative, green=positive, black = neutral) to indicate the sentiment of the respondents' comments.
-
Lack of transparency and explainability: 10-
-
Non-adaptable to my style: 4 –
-
Incorrect suggestions (extreme settings were applied to the plugin, inappropriate cuts): 8-
-
Users appreciated the help of AI, but they said that it is not a substitute for using their ears to assess the quality of the processing applied to the sound: 3
-
Control and ability to correct suggested settings when wrong: 6+, 2-
-
Using smartEQ speeds up my workflow: 11+, 1-
-
Educative 1+
-
Feeling more confident: 1+
The results show that a percentage of the consumers would appreciate more transparency and would like to better understand how the AI derives its parameter suggestions. Additionally, they felt that in some cases, the suggestions for processing the sound sometimes were not optimal, but they valued being able to rectify the errors. Additionally, the consumers thought that using AI had a positive impact by speeding up their workflows. These findings are very much in agreement with some of the key outcomes of the remote usability study I ran, please see the SMART EQ UX STUDY page of my portfolio.
Thematic analysis of open questions
Data analysis of the attitudes and experiences of 159 consumers who have not used AI-assisted equalisers
The figures below show the overall distribution of the consumers' responses to the Likert type questions of the questionnaire.

Here is a list of the questions that consumers were asked:
-
Importance of explainability = It is important to me to understand how the assistive equalizer derives the recommended parameter settings.
-
Positivity towards trying a SmartEQ = I want to try an assistive equalizer.
-
Anticipated productivity boost = I think that assistive equalizers could speed up my workflow.
-
The anticipated increase in confidence = I would feel more confident applying equalization if I used an assistive equalizer that suggests relevant settings by analyzing the sound I want to process.
-
Anticipated usefulness = An assistive equalizer would be very useful to me.
-
Unlikely to trust = I would not trust the suggestions of the assistive equalizer.
-
Positive word of mouth = I heard positive comments from others about assistive equalizers.
1 = strongly disagree, 5 = strongly agree
A general ANOVA model was computed to investigate the effect of the users' age, skill type, skill level, and income factors on the consumers' feelings, trust, and perceived product quality metrics reported by the participants. An asterisk and green highlight color (*) indicate statistical significance.

Post-hoc testing, blue highlighted fields in the image shows which factor levels contribute to the observed effects.

Results
For those participants who have tried assistive equalisers before, we saw a strong consensus among the respondents for wanting to understand how the model derives its recommendations. Looking at the quantitative data for the variable Explanability, 79% of participants said knowing how the AI derives the recommended parameter settings is important. The desire for understandable and transparent AI recommendations was not affected by the participants' age, skill type, or level of expertise. The qualitative data confirm these findings, as multiple users did not like the lack of transparency and explainability in the EQ they had tried, as suggested by the user comments.
-
"Would love some explanations of what is corrected by AI and why."
-
"There is no particular informative reasoning provided for the recommendation. 'Black Box' of secret sauce."
-
"The "why" this setting was chosen. An AI explaining the decision made would be useful. Even if I can guess it from the settings".
Based on the quantitative data on the Net Promoter Score metric, the majority of the users would happily recommend the assistive equalisers to a friend or colleague. This result suggests that participants have a positive attitude towards this technology and thought it could add value to their existing workflow. This interpretation is further supported by the fact that 62% of the participants agreed that using the assistive EQ increased their productivity, and 55% found using the Assistive EQ useful. Below are some comments derived from the qualitative data that confirm the reasons participants responded positively to these questions:
-
"Speeding up workflow and sometimes correcting things I might not."
-
"The speed to use as a starting point when equalising"
-
"I like the velocity of workflow."
-
"The speed to use as a starting point when equalising"
The qualitative data also give us insights into why participants responded neutrally or negatively to some questions. Our interpretation and findings show that there are three critical reasons for the neutral and negative responses that are supported by the user comments below:
Topic 1: the AI does not adapt to the users' style
-
"Personal taste is very important, auto eq doesn't work in this way."
-
"Too much "mystic" and often not adjusted to my own music style"
-
"it's impossible to get the assistive equaliser to give you the exact sound you are looking for."
Topic 2: it makes bad suggestions at times
-
"It doesn't respect the material by going too far in its settings."
-
"I have got some good results on single instruments, but when I applied it to a mix, the results were disappointing."
-
"Frequency bands and amount of gain applied are sometimes completely missing."
and
Topic 3: it can be time-consuming to rectify the errors
-
It's quicker and easier to set things manually than it is to wrestle the AI.
-
Sometimes it is impossible to tweak the recommended settings, so I resort to other EQ plug-ins.
The above response might also help explain the neutral and negative responses we received about the other three UX metrics, feeling in control, Smart EQ performance, and making participants feel more confident in applying EQ.
Given that users, when processing sound using equalisation make subjective and creative decisions that take multiple variables into account, such as stylistic (e.g., music genre) and the musical context (i.e., how the sound fits in the overall mix) and the AI equalisers cannot take these aspects into account yet, giving multiple eq settings suggestions to the user to choose from and giving complete control to customise AI suggestions and rectify errors is very import. Additionally, applying the suggestions to the regular traditional EQ, with a familiar user interface (e.g., EQ graph), is essential for maintaining consistency with existing users' mental models making the technology easier to use and increasing its usefulness. Violating these factors could negatively impact user acceptance, usefulness, and the adoption and success of the product. The qualitative data show that many positive comments were related to the controllability of the Assistive EQ, for instance.
User comments suggesting the importance and appreciation of controllability:
-
Since most are suggestive, I can tweak the settings to taste. It's quite useful, indeed.
-
I can tweak the settings to taste.
-
The ability to have a valid starting point for my manipulation of the sound.
-
I like picking the right section of music where it scans and bases the settings from.
-
Being able to reference other mixes and suggest settings for my own mix.
-
They were perfect 9/10 times, just requiring a little tweaking in some cases.
The hypothesis tests we ran on the participants' quantitative data for the UX metric feeling more confident; we saw that amateur users are way more likely to feel more competent and confident using an AI-assisted equaliser than advanced users. Regarding the UX metric Trust, measured by the Likert statement, "I trust the suggestions of assistive equalisers which I have tried", we found that musicians are significantly more likely to use the EQ suggestion than engineers. Moreover, the data suggest that creative users are significantly more likely to be influenced by AI suggestions than engineers. Finally, amateur users would be more likely to recommend AI-assisted EQ to a friend or colleague than advanced users. These findings do not surprise me and, in a way, confirm our pre-existing assumptions. Essentially, the group differences we were able to measure and reveal through the statistical tests are because engineers and advanced users are more likely to be competent and skilful in using EQs than musicians, creatives, and amateur users. Hence, the engineers and advanced users' competency makes them less likely to trust or accept the parameter settings of the AI. Consequently, these user groups are less likely to recommend such products to friends or colleagues.
For those participants who have not tried an assistive equaliser, we saw a strong consensus among the respondents for wanting to understand how the model derives its recommendation. Looking at the quantitative data for the variable Explanability, 79% of participants said knowing how the AI derives the recommended parameter settings is important to them. The ANOVA analysis model showed significant differences regarding the effect explainability as a result of the participants' skill type, expertise, and age. Posthoc tests revealed that beginners and musicians care less about explainability than professional and engineer consumers. The majority of the consumers ( i.e. 71%) who had not tried an AI-assisted equaliser are positive about using such a tool. The most prominent anticipated benefits of using such tools are improved productivity and increased confidence levels that the equalisation setting they applied to process the sound is appropriate. These benefits are shared by all participants irrespective of their skill level or type of expertise. A large percentage (46%) reports that they would most likely trust the AI recommendation.
Data analysis of 261 consumers' attitudes towards AI in music production
The figures below show the overall distribution of the consumers' responses to the Likert-scale questions of the questionnaire.

Here is a list of the questions that consumers were asked:
-
AI Empowerment =Human and AI co-creation empower sound and music practitioners.
-
Interested in SmartFX = I am very interested in using AI-augmented audio effects in my audio production.
-
AI will improve how we process sound = AI-augmented audio effects will change how we process sound for the better.
-
Adaptability =It is very important that AI-assisted effects learn and adapt over time to my style.
-
Optimism of the potential of AI capabilities = Sound and music AI technologies will reach levels of performance that will be indistinguishable from human experts.
-
Fear that AI will affect the audio job market = Creative applications of AI threaten sound and music professionals' career prospects.
-
Trust more humans than AI = I don't like relying on AI for creative activities and prefer to trust human experts.
1 = strongly disagree, 6 = strongly agree
A general ANOVA model was computed to investigate the effect of the users' age, skill type, skill level, and income factors on the consumers' attitudes towards AI. An asterisk and green highlight color (*) indicate statistical significance.
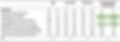
Post-hoc testing, blue highlighted fields in the image shows which factor levels contribute to the observed effects.

Outcomes

The results of this study show that the consumer market is ready to accept AI tools for music production regardless of the consumer type of skill (i.e., creative, musician, engineer), or their skill level (i.e., amateur, professional, advanced users), or their age. People express optimism about the potential of AI-augmented audio effects to improve existing user workflows. Moreover, the majority of respondents believe that in the future, sound and music AI technologies will reach levels of performance that will be indistinguishable from human experts. However, respondents' opinions are more divided on whether AI will positively or negatively impact the audio job market. Furthermore, given the current state of maturity of the technology, respondents were also divided about whether they would be inclined to rely on and trust more AI technologies over skilled human professionals.
Based on the current state of the technology, the customer segments that are more likely to benefit from adopting the AI equalisers are beginners, amateur creative and musician users who are less skilled than professionals and engineers whose competency level in using equalisers is high. This is a valuable insight for determining the most potent tentative customers for this type of product, taking into account the current maturity of the technology. The appeal will likely extend to all customer segments as the technology improves. Moreover, these insights are potentially informative for marketing purposes, such as shaping marking content, strategic planning, product placements, and value proposition development.
The findings of this study also showed that all customer segments would wish to understand why AI is making particular recommendations. However, for advanced and professional users, explainability, transparency, and adaptability of AI are important factors that will aid in accelerating the adoption of AI technology. These insights are informative for product teams touching on both disciplines user experience design and machine learning. The relevant questions for the ML engineers are how to develop models or systems that can provide sufficient information to the user with regards to why the AI made certain decisions and what problems the AI identified and tried to solve. Furthermore, which methods should be utilised to allow the machine learning model to adapt to the user's style over time and learn from the users' behaviour? From the UI designers' point of view, the challenge is finding ideal display methods to make this type of contextual information available to the user as an unintrusive layer on top of the existing complex user interface of an equaliser. These raise questions related to representation, e.g., match relevant norms and semantic terms that deliver the information in a way that users expect and understand. Additionally, the level of control of the automation and the ability to correct the AI suggestions when they do not meet the users' expectations are desirable features independent of the users' skills and experience level.
Recommendations

-
Based on the technological maturity of AI-assisted equalisers and that of other audio effects, we identify beginner and amateur users as the most tentative customer segments. Hence, value propositions, market positioning, and branding should ideally target, reach and appeal to these segments. As technology improves, the professional and engineering customer segments will also become tentative.
-
Critical benefits for those customer segments are improved confidence in their ability to apply equalisations for processing sound and speeding up their workflows.
-
Since most AI models currently fail always to provide good results and take into account stylistic, personal taste, and contextual information about the musical context, the user interface must not remove the users' ability to dismiss and correct the AI recommendations.
-
Additionally, offering multiple sound processing recommendations will help maximise the chances of providing users with useful results that match their preferences and creative and aesthetic vision.
-
The ability to provide relevant contextual information to the user that would help them understand why the AI model suggests the parameter settings to the user can improve the perceived levels of trust and increase the adoption of the technology by all customer segments but, most importantly, by advanced and professional users.
-
Based on the findings of this study, many people have not yet tried using AI in their music production workflow, but they are very optimistic about using such technologies. This suggests that the market is far from being saturated. With the current hype about AI technologies (ChatGPT, Dall-E), there is a strong business case and the right time to invest in AI-augmented audio products.